Keynote Speakers
We are very pleased to have acquired the services of an excellent selection of keynote speakers for the symposium The speakers and the titles of their talks are shown below.
Prof Monica Bianchini
University of Siena, ItalyModeling biological processes with Graph Neural Networks
More details ...
Prof Xiaopeng Li
University of South Florida, USAOperations and Planning for Connected and Automated Transportation Systems
More details ...
Prof Sheela Ramanna
University of Winnipeg, CanadaDeep Neural Network Applications: Production of Real-time Road Conditions and Land-Use/Land-Cover Maps
More details ...
Prof Kenji Suzuki
Tokyo Institute of Technology, JapanArtificial intelligence for medical image diagnosis
More details ...
Prof Monica Bianchini
University of Siena, ItalyModeling biological processes with Graph Neural Networks
Abstract:
Major advances in high-throughput technologies have recently produced an enormous amount of molecular data, making them available for in silico analysis. Indeed, DNA, RNA, proteins and metabolites play a crucial role in the molecular mechanisms at the basis of the cellular processes underlying life. Therefore, studying their structure and interactions is crucial in a variety of applications, ranging from the development of new drugs to the discovery of disease pathways. Since graphs provide a simple and intuitive representation of heterogeneous and complex biological processes, the modeling and understanding of complicated molecular mechanisms can be based on the use of graph theory and machine learning techniques for graphs. Graph-based neural networks are a powerful tool for managing graphs, and interest in their study and applications has been explosive in recent years. In my talk, I will review the history of GNNs from their rise to current models, while also exploring their applications for generating molecular graphs for drug discovery, predicting protein-protein interfaces, and integrating multi-omics data.
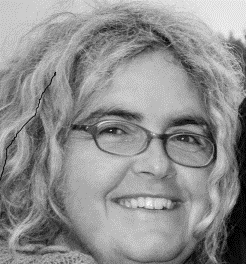
Biography:
Monica Bianchini received the Laurea degree cum laude in Applied Mathematics in 1989 and the Ph.D. degree in Computer Science and Control Systems in 1995 from the University of Florence. She is currently an Associate Professor at the Department of Information Engineering and Mathematics of the University of Siena. Her main research interests are in the field of machine learning, with emphasis on neural networks for structured data and deep learning, approximation theory, bioinformatics, and image processing. M. Bianchini has authored more than one hundred papers and has been the editor of books and special issues on international journals in her research field. She has been involved in the organization of several scientific events, including the NATO Advanced Workshop on Limitations and Future Trends in Neural Computation (2001), the 8th AI*IA Conference (2002), GIRPR 2012, the 25th International Symposium on Logic-Based Program Synthesis and Transformation, and the ACM International Conference on Computing Frontiers 2017. Prof. Bianchini served/serves as an Associate Editor for IEEE Transactions on Neural Networks (2003-09), Neurocomputing (from 2003), and Int. J. of Computers in Healthcare (from 2010). She is a permanent member of the Editorial Board of IJCNN, ICANN, ICPR, ICPRAM, ESANN, ANNPR and KES.
Prof Xiaopeng Li
University of South Florida, USAOperations and Planning for Connected and Automated Transportation Systems
Abstract:
Advanced connected and automated vehicle (CAV) technologies can be utilized to achieve precise vehicle trajectory control and render unprecedented opportunities to improve transportation system performance in safety, mobility and sustainability. However, emergency of CAV technologies brought significant challenges to traffic operators and transportation planners in understanding, managing and planning for CAV traffic, particularly when mixed with regular human driven vehicles. This presentation will introduce theories, models and empirical findings of CAV traffic via modeling and experiments, ranging from microscopic trajectory control to macroscopic traffic capacity. Opportunities and challenges from individual CAV control to collective traffic management will be presented. How to accommodate CAV traffic in traffic operation practice and transportation planning activities will be discussed.
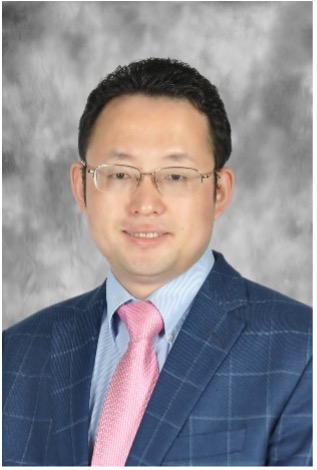
Biography:
Dr. Xiaopeng (Shaw) Li is currently an associate professor in the Department of Civil and Environmental Engineering at the University of South Florida (USF). He is the director for one USDOT national university transportation center, National Institute for Congestion Reduction (NICR). He established the Connected and Automated Transportation Systems Lab that houses two L3 connected automated vehicles equipped with the USDOT CARMA platform. He is the first holder of Susan A. Bracken Faculty Fellowship at USF and is a recipient of a National Science Foundation (NSF) CAREER award. His major research interests include automated vehicle traffic control and connected & interdependent infrastructure systems. He has served as the PI or a co-PI for a number of federal (NSF, USDOT, USDOE), local (e.g., state DOTs, UTCs, I-4 Corridor Program) and industry grants, amounting to a total budget around $15 million. He has published over 70 peer-reviewed journal papers, many of which are in top journals such as Transportation Research Part B, Transportation Science and Operations Research. He has served as a member on the Transportation Network Modeling Committee (ADB30) and the Traffic Flow Theory and Characteristics (AHB45) of the Transportation Research Board (TRB) and an Associate Editor for IIE Transactions and have also served on the editorial boards for Transportation Research Part B, Part C, Part E, the ASCE-ASME Journal of Risk and Uncertainty in Engineering Systems, etc. Dr. Li received a B.S. degree (2006) in civil engineering with a computer engineering minor from Tsinghua University, China, a M.S. degree (2007) and a Ph.D. (2011) degree in civil engineering along with a M.S. degree (2010) in applied mathematics from the University of Illinois at Urban-Champaign, USA. Please check http://ce.eng.usf.edu/facultyandstaff/xiaopengLi.htm for more information.
Prof Sheela Ramanna
University of Winnipeg, CanadaDeep Neural Network Applications: Production of Real-time Road Conditions and Land-Use/Land-Cover Maps
Abstract:
In this talk, we present two applications of Deep Neural Networks: i) in Intelligent Transportation with Real-time Road Condition Map and, ii) in large-scale monitoring of land resource changes with Land-Use/Land-Cover Maps.
Road Weather Information Systems (RWIS) can provide real-time road weather information at point locations, which is often used to produce road weather forecasts. Since cameras are more prevalent than RWIS, the extraction of road conditions data, can provide a source of new observational data which can be assimilated into pavement forecast models, which normally use RWIS data as an input. Therefore, it is of value from both a monitoring and forecasting perspective to quickly extract road conditions data on a large scale. We present results obtained via leveraging state-of-the-art convolutional neural networks in labelling images taken by street and highway cameras located across North America. The classified images are then used to construct a map showing real-time road conditions at various camera locations across North America.
The second part of the talk focuses on an application of deep neural networks approach to Land use and Land cover (LULC) mapping from Landsat 5/7 multispectral satellite images taken of the Province of Manitoba in Canada. We show that by classifying each pixel in a satellite image into a number of LULC categories, we are able to successfully produce LULC maps. The presented solution is increasingly important since the abundance and affordability of satellite imagery has led to many government and private industries using LULC maps as a fundamental tool for large-scale monitoring of land resource changes.
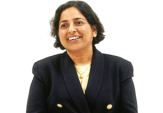
Biography:
Dr. Sheela Ramanna is a Full Professor and past Chair of the Applied Computer Science Department. She is the co-founder of the ACS graduate studies program University of Winnipeg. She received a Ph.D. in Computer Science from Kansas State University, U.S.A and a BS in Electrical Engineering and MS in Computer Science and Engineering from Osmania University, India. She serves on the Editorial Board of Springer Transactions on Rough Sets (TRS) Journal, Elsevier Engineering Applications of AI Journal, KES Journal and Advisory Board of the International Journal of Rough Sets and Data Analysis. She is the Managing Editor of the TRS and is a Senior Member of the IRSS (Intl. Rough Set Society). She has co-edited a book with L. C Jain and R. Howlett on Emerging Paradigms in Machine Learning published in 2013 by Springer. She has served as Program Co-Chair for MIWAI 2013, RSKT 2011, RSCTC 2010 and JRS2007. She is currently the Program Co-Chair of IJCRS 2021 track of IFSA/EUSFLAT 2021. She is the recipient of a TUBITAK Fellowship (Turkey) for 2015. Her research is funded by Natural Sciences and Engineering Research Council of Canada Discovery and Engage Grants Program. She has received more than $1,130,000 in research funding since 1992. She has published over 50 peer-reviewed articles in the past 6 years in journals such as Pattern Recognition Letters, Knowledge-Based Systems, Neural Computing and Applications, Granular Computing, Knowledge and Information Systems, Intelligent Information Systems, Mathematics in Computer Science, Frontiers Advances in Computational Neurosciences, and Neuroscience Letters. The focus of her research is in fundamental and applied research in machine learning and granular computing. Her current interests include: i) Tolerance-based granular computing techniques (fuzzy sets, rough sets and near sets) with applications in social networks, natural language processing, computer vision and audio signal processing, ii) topological data analysis, iii) application of descriptive proximities, and iv) deep learning applications.
Prof Kenji Suzuki
Tokyo Institute of Technology, JapanArtificial intelligence for medical image diagnosis
Abstract:
Deep learning in artificial intelligence (AI) has become one of the most active areas of research in the biomedical imaging field including medical image analysis and computer-aided diagnosis (CAD), because "learning from examples or data" is crucial to handling a large amount of data ("Big data"") coming from medical imaging systems. Deep learning, including our original massive-training artificial neural networks (MTANNs), is an end-to-end machine learning model that enables a direct mapping from the input images to the desired outputs, eliminating the need for handcrafted features in feature-based machine learning. Deep learning is a versatile, powerful framework that can acquire medical image-processing and analysis functions through training with image examples. In this talk, deep learning in medical imaging and computer-aided diagnosis is overviewed, including 1) CAD for lung cancer detection in chest radiography and thoracic CT, 2) distinction between benign and malignant nodules in CT, 3) polyp detection and classification in CT colonography in colorectal cancer screening, 4) separation of bones from soft tissue in chest radiographs, and 5) radiation dose reduction in CT and mammography.
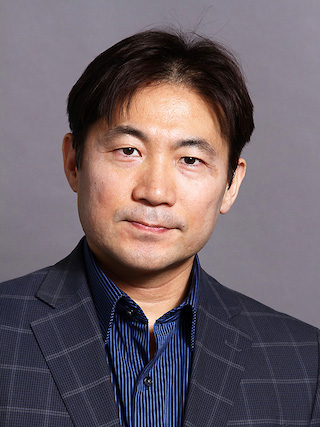
Biography:
Kenji Suzuki, Ph.D, has been actively researching deep learning in medical imaging and AI-aided diagnosis for over 25 years. Prior faculty experiences include University of Chicago and Illinois Institute of Technology. He has published 14 books and over 340 papers and is an inventor on a dozen of licensed and commercialized patents, including one of the earliest deep learning patents. He has been awarded numerous grants, including grants from NIH, NEDO, and JST, chaired 98 international conferences, and served as editor of over 40 leading international journals. Dr. Suzuki has been Professor at Institute of Innovative Research at Tokyo Institute of Technology, where he explores the possibilities of explainable AI.